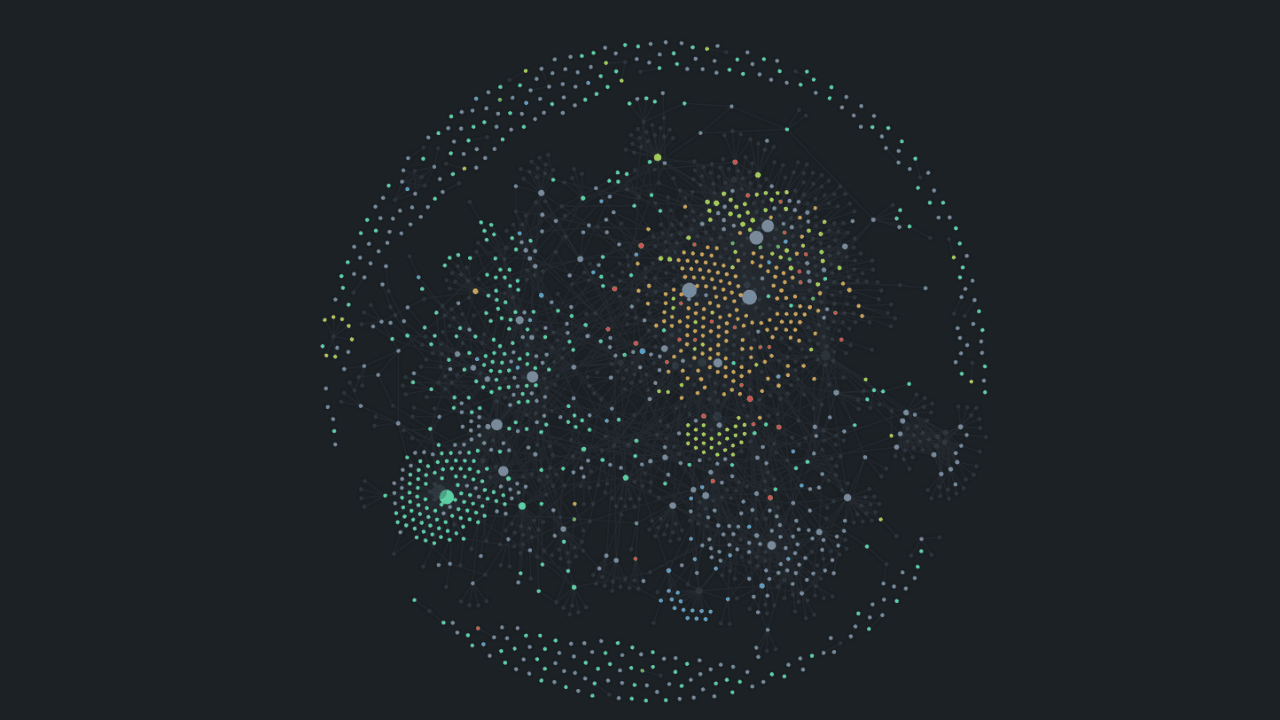
Introduction to AI-Powered Knowledge Graphs and GraphRAG for Business Solutions

Introduction to AI-Powered Knowledge Graphs and GraphRAG for Business Solutions
In today's data-driven business landscape, organizations face a growing challenge: how to effectively manage, connect, and utilize vast amounts of information. AI-powered knowledge graphs are emerging as a revolutionary solution, offering unprecedented capabilities in information retrieval and understanding. Furthermore, recent advancements like GraphRAG (Retrieval Augmented Generation) are taking this a step further, enabling systems to answer more complex, inferential questions by understanding the intricate relationships within data. Let's explore how this evolving technology is transforming business operations.
What is an AI-Powered Knowledge Graph?
A knowledge graph is a structured network of information that represents relationships between different pieces of data. When enhanced with AI, these graphs become dynamic, intelligent systems that can:
- Automatically identify and create connections between concepts
- Learn from new information and user interactions
- Understand context and nuance in queries
- Scale efficiently with growing data volumes
The Rise of GraphRAG: Enhancing Knowledge Graphs
While traditional AI-powered knowledge graphs offer significant advantages, a new approach called GraphRAG is emerging as a game-changer. GraphRAG leverages the power of knowledge graphs to improve retrieval and generation in language models, overcoming some limitations of standard Retrieval Augmented Generation (RAG) techniques.
Baseline RAG systems often rely on retrieving documents based on semantic similarity, which can struggle when the answer requires connecting information across multiple documents or inferring relationships not explicitly stated. GraphRAG addresses this by:
- Automating Knowledge Graph Construction: Utilizing Large Language Models (LLMs) to automatically build knowledge graphs from text documents, making the technology more accessible.
- Leveraging Graph Structure for Retrieval: Instead of just relying on semantic similarity, GraphRAG can traverse the relationships within the knowledge graph to retrieve more contextually relevant information.
- Answering Complex, Inferential Questions: GraphRAG excels at answering questions that require synthesizing information from different parts of the knowledge base, identifying connections and drawing conclusions. For example, in a dataset of earnings calls, it can answer questions like "Which companies are investing in AI?" even if that information isn't explicitly stated in a single document.
Enhanced Retrieval Accuracy
Traditional search systems often rely on keyword matching, leading to limited results. AI-powered knowledge graphs, especially when combined with GraphRAG techniques, revolutionize retrieval accuracy through:
- Contextual Understanding: The system comprehends the intent behind queries, not just the words used, and can understand the relationships between entities mentioned.
- Semantic Search: Results are based on meaning rather than exact text matches, and GraphRAG extends this by understanding the connections between semantically different but related concepts.
- Learning from Interactions: The system improves accuracy over time based on user behavior and feedback.
- Inferential Retrieval: GraphRAG can retrieve information that isn't explicitly stated but can be inferred from the relationships within the knowledge graph.
Advanced Referencing Functionality
One of the most powerful features of AI-powered knowledge graphs is their ability to maintain complex reference structures:
- Bi-directional References: Every piece of information can be both a source and a reference point.
- Version Control: Track changes and maintain historical accuracy of information.
- Authority Ranking: Automatically identify and prioritize authoritative sources.
Broad-Based Understanding and Concept Connection
AI-powered knowledge graphs excel at connecting seemingly unrelated concepts, offering:
- Cross-Domain Insights: Identify valuable connections across different business areas. GraphRAG further enhances this by uncovering non-obvious relationships.
- Pattern Recognition: Discover hidden relationships and trends in data. GraphRAG's graph traversal capabilities make this more efficient.
- Contextual Relevance: Understand how different pieces of information relate to specific business contexts.
As the deepset.ai article highlights, GraphRAG can transform a collection of separate documents into an interconnected web of knowledge, revealing the underlying structure of information for a deeper understanding and more effective analysis. This is particularly useful in domains with complex relationships, such as financial analysis, legal document review, and medical research.
Analytics and Understanding
The analytical capabilities of AI-powered knowledge graphs provide unprecedented insights:
- Usage Patterns: Track how information is accessed and utilized across the organization.
- Impact Analysis: Measure the influence of specific content on business outcomes.
- Knowledge Gaps: Identify areas where information is missing or needs enhancement.
Infinite Scalability
Unlike traditional databases, AI-powered knowledge graphs offer near-infinite scalability:
- Dynamic Growth: Automatically accommodate new information without performance degradation.
- Efficient Processing: Maintain quick response times even with massive data volumes.
- Intelligent Pruning: Automatically archive or remove outdated or irrelevant information.
Real-World Applications, Including GraphRAG
At Cypress AI, we've implemented knowledge graphs, and are exploring GraphRAG techniques, to revolutionize processes like RFP responses:
- Smart Content Retrieval: Instantly find relevant past responses and supporting documentation. GraphRAG can improve this by understanding the relationships between different RFP sections and your capabilities.
- Relationship Mapping: Understand how different RFP requirements relate to your organization's capabilities. GraphRAG excels at this by explicitly mapping these connections.
- Continuous Learning: Improve response quality by learning from successful proposals.
As the article on why GraphRAG is hard to get right points out, internal document datasets are a perfect use case for graph-based approaches. The ability to connect people, projects, products, and customer information within an organization makes GraphRAG a powerful tool for enhancing knowledge sharing and retrieval.
Getting Started with AI-Powered Knowledge Graphs and GraphRAG
Implementing an AI-powered knowledge graph, and potentially incorporating GraphRAG techniques, might seem daunting, but with the right partner, the transition can be smooth and impactful. The key is to:
- Start with a specific use case. Consider areas where connecting disparate information is crucial.
- Focus on data quality over quantity. A well-structured knowledge graph is more valuable than a large, disorganized one.
- Build incrementally. Start with core entities and relationships and expand over time.
- Measure and adjust based on results. Track the effectiveness of your knowledge graph in improving information retrieval and insights.
Ready to explore how AI-powered knowledge graphs and GraphRAG can transform your business? Schedule a demo to see Cypress AI's knowledge graph technology in action.